The Puget Sound Institute is facilitating a series of online workshops and discussions to explore the technical uncertainties related to the science of Puget Sound water quality. As part of the project, we are publishing informational blogs and articles, including this look at how computer models are becoming increasingly important to our understanding of the natural world. The project is jointly sponsored by King County and the Puget Sound Institute.
By Christopher Dunagan
If you are planning a hike, picnic or other outdoor activity, it might be wise to take a look at the local weather forecast. Thanks to advanced computer modeling and data-gathering equipment, the National Weather Service has gotten pretty good at predicting rain or shine for the coming hours and days. A five-day forecast is about 90 percent accurate, according to the Weather Service.
Mathematical models running on high-speed computers are being used successfully to describe and predict all sorts of environmental changes, from annual salmon runs to sea-level rise to warming waters in streams, bays and oceans. The Salish Sea Model, valuable in assessing water quality in Puget Sound, could become a key tool in setting regulatory limits for nitrogen discharges from sewage-treatment plants and other nitrogen sources around Puget Sound.
In writing scientific articles, I often find myself referring to predictions derived from all sorts of models. Rarely do I delve into the workings of these models to understand how they function or to consider their reliability; I let the experts do that. But since models are being used more and more to help determine environmental policies, I would like to take a step back and consider what models are all about.
With help from six experts involved in a variety of ecosystem models, I’ve come up with a list of very basic things that everyone should know about modeling. In the future, I plan to discuss several models being used in the Puget Sound region and how they facilitate scientific discovery while helping political leaders make critical decisions.
Basic things to know about modeling:
Some models are complex, but others are quite simple. At a basic level, most people rely on mental models to make everyday decisions.
“People may feel that models are mysterious, but they are just a way for people to better understand the world,” said Mindy Roberts, an environmental engineer who helped develop various ecosystem models for the Washington Department of Ecology before joining the Washington Environmental Council.
For example, she said, when driving to work or the store, one route may be shorter but another route may be faster depending on conditions. One roadway may be more congested at certain times of the day or on certain days of the week. Using knowledge of the alternate routes to reach a conclusion is basic modeling.
If the goal is to save time, you might consider the various traffic conditions that could slow you down. If cost is important, you might take the shorter but slower route to save on gasoline, or maybe a longer route could avoid tolls. Perhaps a mass-transit option would work for you. Along with other factors, this process of weighing the various options is a form of modeling.
On a given day, your immediate decision might be to just take the fastest or shortest route, given the conditions. But if the budget is tight, you might want to calculate the costs in time and money of various options over the next month or year. With that, you would be getting into more advanced modeling with the use of basic math.
Ecosystem models are simplified representations of complex systems found in nature. Models allow for virtual experiments that would not be possible in the real world.
Models are based on an understanding of natural processes — physical, chemical and biological. Changes in the natural system are expressed through mathematical formulas. Wondrously, nature’s complexity exists far beyond anything that can be replicated by even the most sophisticated models — even assuming that one could understand each and every process taking place. Modeling involves making important choices about which processes to include and which to leave out of the calculations.
A successful model will not only reflect conditions and changes that can be actually measured in the real world, it will be able make predictions for any time and place within the parameters set for the model — often well into the future. Climate models, for example, may present a graph of global temperatures over the next 50 years, based on the best available information about the effects of various greenhouse gases on natural systems.
Parker MacCready, a research professor and physical oceanographer at the University of Washington, works on models of coastal and estuarine systems. The sophisticated LiveOcean model provides three-day forecasts of conditions off the West Coast, including currents, temperature, salinity and even harmful algal blooms. The model updates each day, taking new inputs of information about the state of the ocean, atmosphere and rivers, to produce something like a weather forecast with maps of expected ocean conditions.
“The most frequent misconception I encounter,” MacCready said, “is when I show someone some model output, like a surface salinity map, and they ask, ‘Where did you get all that data.’ I have to explain what a model is and how we use the very limited data available for testing and forcing.”
In other words, salinity levels represented by numbers on a map are the model’s projections for a specific time and place. Data — actual measurements — are used to build and drive the model and to determine how successful the projections turn out to be. In the end, the accuracy of those projections is the most important thing.
To press the point, MacCready suggests envisioning a model for baking a cake. The known data — actual information — is the temperature of the oven, which closely resembles the surface temperature of the cake. Mathematical expressions can be written for how fast heat penetrates through the cake, causing the cake batter to solidify and eventually be ready to eat. One can gather data along the way by checking the inside stickiness with a toothpick, he noted. But if the model performs well, the cake will be done through the middle and can be removed from the oven before the outside gets burned.
Models are designed to answer specific questions. Modelers strive to use the simplest model that can answer the question with the available information.
“I really like thinking of models as tools, and you have different models for different needs,” said Jan Newton, senior principal oceanographer at the UW’s Applied Physics Laboratory and co-director of the Washington Ocean Acidification Center. “Hammers and screwdrivers have different applications. You can’t say that one model will answer all of our questions.”
The “right” model will not only reflect dynamic changes in the natural world, but its weaknesses and assumptions will be known and explicitly stated. Most important, Newton stressed, is the need for validation by comparing the model’s predictions to real-world observations, a process also known as “skill assessment.”
Because models are less-than-complete representations of natural systems, every model will have uncertainty, she said. “The question is figuring out if the uncertainty is at an acceptable level. A skill assessment helps you understand to what degree you can trust the model.”
Another form of validation is assigning different models to the same problem and seeing how close they come to the same answer, she said.
Mike Brett, a UW professor of civil and environmental engineering, says the Achilles heel of modeling efforts is often the lack of information about the real world, such that the model does not reflect how the natural system actually works. For example, the growth rates of algae based on nutrient levels are almost never known for the particular system being modeled, so they are guesstimated during the model-calibration process. The result may be “data fitting,” he said, an effort to change the model to make it consistent with the data.
You can end up with “the right answers for the wrong reasons,” a phrase sometimes applied to models that don’t work very well, he said. “All modelers have to be worried whether the model is just fitting the data or really representing the system being modeled.”
According to MacCready, models should be kept as simple as possible for the job at hand. Adding new processes to simulate ever more natural functions increases time and resources — including costly time on super computers needed to derive model outputs, the answers you are seeking.
“When you add more processes, you can rapidly run out of your ability to test the model,” MacCready said, adding that he has plenty of data about temperature and salinity for his model but much less about currents and even less about the growth rate of plankton needed to predict algal blooms.
A model may never truly be “finished,” because it always raises new questions to be explored, but the model may be good enough to answer the question being posed at the moment.
“It is a widely held myth that a model cannot be developed before we have sufficient data and a comprehensive understanding of the system,” says ecological modelers Steven Railsback and Volker Grimm in their 2013 book on modeling. “The opposite is true; our knowledge and understanding are always incomplete and this, exactly, is the reason to develop models.”
Constraints on information, understanding or time are painful, they argue, but if one develops a clear definition of the problem to be addressed by the model, then the modeler will have a guidepost for when to stop.
Not all modelers I interviewed agreed with the idea that a model is never finished, but most agreed that time and resources constrain the work that can be done, both in modeling and in gathering real-world data.
“There is a level of diminishing returns,” said Roberts. “The question becomes ‘What is the level of uncertainty and what can we do about it?’”
The methods of modeling have undergone a technical and systemic evolution, noted Stefano Mazzilli, senior research scientist at Puget Sound Institute. One model builds upon the findings of another. In fact, in coupled models, the outputs of one model become the input of another model. Also, smaller models (called modules) may be integrated into larger models — such as the “sediment diagenesis module” used in the Salish Sea Model to better characterize sediment biochemistry that influences oxygen levels in waters of Puget Sound.
Modeling is a collaborative process
Most models are built upon the framework of previous models designed elsewhere in the world and altered, as needed, to local conditions. In designing a model for a specific purpose, collaboration comes into play in a variety of ways, according to Ben Cope, an environmental engineer for the Seattle Regional Office of the Environmental Protection Agency.
Basic processes and parameters reflected in the model may come from studies in nature or in a laboratory. The ideas may be part of the scientific literature, or they could be shared directly by experts working in a specific discipline, such as hydrodynamics or plankton biology, Cope noted. Modelers may ask various experts if their assumptions seem reasonable as the model goes through development.
Where the model involves a specific location, such as a stream or inlet, local residents may be approached to answer questions that might identify unique local conditions, such as how a dam or a streambed affects currents, Cope said.
Peer review by fellow modelers and other scientists often takes place at several levels, he said, from informal sharing to review for publication. “You get a lot of people looking at the model to make sure you are on track.”
The inner workings of a model, including the computer code that runs it, may or may not be revealed, depending on a model’s ownership and use and who needs to see it, Cope said. On the other hand, when a model is being used to make regulatory decisions, everything is laid upon the table. The information is provided publicly through a “detailed model development report,” which is subject to public scrutiny during the review process for proposed regulations.
Because of the nature of the scientific process and peer review, modelers have a strong incentive to make sure their models are the best representations of the natural world.
“Modelers more than anyone want to get the models right,” Cope said. “This is a scientific exercise, and transparency is everything.”
Building large-scale models is a difficult task, and the culture of the people involved is to make the model as accurate as possible, he continued. From the beginning concepts for a model through cycles of validation, testing and revision, the work goes on.
“There is going to be error and uncertainty,” he said, “but you address that and eventually you get the model as good as you can do.”
Related:
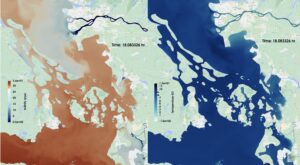
The next online workshop in our Science of Puget Sound Water Quality series will be held next Thursday, September 29th from 8 to 10 a.m. and will focus on water-quality modeling and monitoring data. Registration is required.
The following workshop, on Oct. 6, is titled “Biological Integrity of Key Species and Habitats.” It will feature talks on eutrophication in the Baltic Sea, effects of eutrophication on key species, and modeling tools to address the problem. Registration is required. Information about the workshops and ongoing collaboration efforts can be found on PSI’s website.
The workshop series is funded by King County.